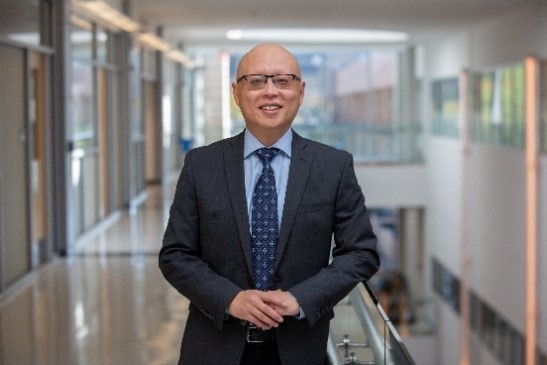
主讲人:殷亚峰
邀请人:杜豫川教授
时间:2023年10月19日(周四)下午13:00-15:00
地点:通达馆103报告厅
主讲人简介:
Dr. Yafeng Yin is a Donald Cleveland Collegiate Professor of Engineering and Donald Malloure Department Chair of Civil and Environmental Engineering at University of Michigan, Ann Arbor. He works on transportation systems analysis and modeling and has published nearly 150 refereed papers in leading academic journals. He currently serves as Area Editor of Transportation Science, and Associate Editor of Transportation Research Part B: Methodological and was the Editor-in-Chief of Transportation Research Part C: Emerging Technologies between 2014 and 2020. Dr. Yin received his Ph.D. from the University of Tokyo, Japan in 2002, his master’s and bachelor’s degrees from Tsinghua University, Beijing, China in 1996 and 1994 respectively. Prior to his current appointment at the University of Michigan, he was a faculty member at University of Florida between 2005 and 2016, a postdoctoral researcher and then assistant research engineer at University of California at Berkeley between 2002 and 2005. Between 1996 and 1999, he was a lecturer at Tsinghua University. Dr. Yin has received recognition from different institutions, including the Monroe-Brown Foundation Education Excellence Award from University of Michigan, a Doctoral Mentoring Award from University of Florida, and Stella Dafermos Best Paper Award, Ryuichi Kitamura Paper Award, and Kikuchi-Karlaftis Best Paper Award from Transportation Research Board.
主讲内容简介:
In this talk, I will share some results from our ongoing research that aims to transform transportation network equilibrium modeling paradigm via end-to-end learning. Our research was motivated by observing that existing equilibrium analysis paradigm has been established via a "bottom-up" assembly approach, in which the selection of behavior model for travel choices or the calibration of the supply function is divorced from the end goal of the model building, i.e., prescribing an equilibrium flow distribution that matches observations as closely as possible. Leveraging recent advancements in game theory and machine learning, we hope to develop an end-to-end framework that directly learns the demand- or/and supply-side components and the equilibrium state from empirical data.
欢迎各位老师、同学参加院级高等讲堂!
tyc8722太阳集团城研究生会
tyc8722太阳集团城青年教师沙龙