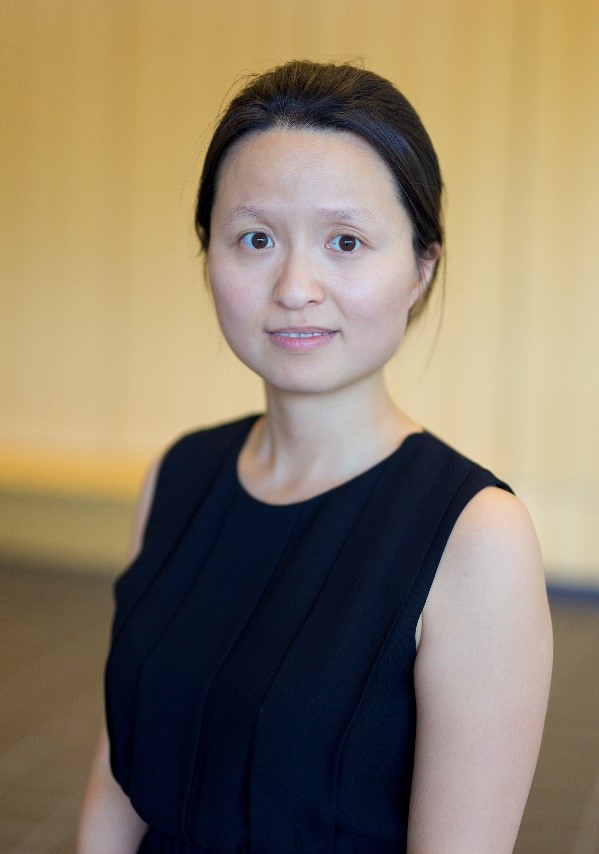
主讲人:Xuan (Sharon) Di Assistant Professor
邀请人:张华
学科方向:交通运输
时间:2019年8月27日(周二)下午13:30
地点:通达馆436
主讲人简介:
Xuan (Sharon) Di is a tenure-track Assistant Professor in the Department of Civil Engineering and Engineering Mechanics at Columbia University in the City of New York since September 2016 and serves on a committee for the Smart Cities Center in the Data Science Institute. Prior to joining Columbia, she was a Postdoctoral Research Fellow at the University of Michigan Transportation Research Institute (UMTRI). She received her Ph.D. degree from the Department of Civil, Environmental, and Geo-Engineering at the University of Minnesota, Twin Cities in 2014. Dr. Di received a number of awards including the Transportation Data Analytics Contest Winner from Transportation Research Board (TRB), the Dafermos Best Paper Award Honorable Mention from the TRB Network Modeling Committee, Outstanding Presentation Award from INFORMS, and the Best Paper Award and Best Graduate Student Scholarship from North-Central Section Institute of Transportation Engineers (ITE). She also serves as the reviewer for a number of journals, including Transportation Science, Transportation Research Part B/C/D, European Journal of Operational Research, Networks and Spatial Economics, and Transportation.
Dr. Di directs the DitecT (Data and innovative technology-driven Transportation) Lab @ Columbia University. Her research interests include emerging transportation systems optimization, shared mobility modeling, and data-driven urban mobility modeling, leveraging optimization, game theory, and data analytics. Details about DitecT Lab and Prof. Sharon Di’s research can be found in the following link: http://sharondi-columbia.wixsite.com/ditectlab.
主讲内容简介:
This talk is composed of two topics. In the first topic, I will introduce a model we developed in collaboration with Didi Chuxing. Vacant taxi drivers’ cruising behavior to seek the next potential passenger in a road network generates additional vehicle traveled miles, adding congestion and pollution into the road network and the environment. This study aims to employ reinforcement learning to model idle e-hailing drivers’ optimal sequential decisions in passenger-seeking. While there exist a few studies that applied Markov decision process (MDP) to taxi drivers searching behavior, these studies were primarily focused on modeling traditional taxi drivers behavior. Transportation network companies (TNC) or e-hailing (e.g., Didi, Uber) drivers exhibit different behaviors from traditional taxi drivers because the e-hailing drivers do not need to actually search passengers. Instead, they reposition themselves so that the matching platform can match a passenger. Accordingly, we incorporate e-hailing drivers' new behavioral features into our model. We then train the model with over 15,000 Didi drivers 1-month trajectories. To validate the effectiveness of the model, a Monte Carlo simulation is conducted to simulate the performance of drivers if they follow optimal policies derived from our model. Two metrics, the rate of return and taxi utilization rate, show that our model can help drivers increase drivers' rate of return by 14% and improve taxi utilization rate by 26%.
My second topic is about selfdriving cars. As this era’s biggest game-changer, autonomous vehicles (AV) are expected to exhibit new driving and travel behaviors, thanks to their sensing, communication, and computational capabilities. However, a majority of studies simply tailor human-driven vehicles (HV)’s behavior for AVs by tweaking some behavioral parameters. In these models, AVs are essentially human drivers but react faster, “see” farther, and “know” the road environment better. We believe AVs’ most disruptive characteristic lies in its intelligent goal-seeking behavior. Accordingly, in this talk, I will first introduce a game-theoretic framework we have developed to model autonomous driving behavior. To illustrate the potential advantages that AVs may bring to stabilize traffic, we propose a multi-class game where AVs are modeled as intelligent game-players and HVs are modeled using a classical non-equilibrium traffic flow model. Numerical results are provided to illustrate that a certain portion of AVs can stabilize traffic.
欢迎各位老师、同学参加同路人学术论坛!
tyc8722太阳集团城研究生会
tyc8722太阳集团城青年教师沙龙